- FuturePoint Digital AI Blog
- Posts
- The Power of Leveraging AI for Interdisciplinary Research and Discovery: Material Science and Medicine
The Power of Leveraging AI for Interdisciplinary Research and Discovery: Material Science and Medicine
Our multi-part series on AI & frame-breaking discoveries...

In the highly dynamic worlds of science and technology, the convergence of diverse fields is driving groundbreaking innovations. One such intersection is between material science and medicine, where the integration of AI is revolutionizing the development of biocompatible materials for medical implants. By harnessing the predictive power of machine learning algorithms, researchers can accelerate the discovery and optimization of new materials tailored for healthcare applications. This blog explores how AI is bridging the gap between material science and medicine, highlighting key examples and case studies that demonstrate the potential of this interdisciplinary approach.
The Convergence of Material Science and Medicine
Material science focuses on the study of the properties, composition, and applications of materials (Britannica, n.d.), while medicine aims to improve human health through diagnosis, treatment, and prevention of diseases (Medical News Today, 2018). The intersection of these fields, powered by AI, is fostering the creation of advanced biocompatible materials that are crucial for medical implants such as prosthetics, stents, and tissue scaffolds (MDPI, 2023).
The Role of AI in Accelerating Material Discovery
AI, particularly machine learning, plays a pivotal role in expediting the research process by analyzing vast datasets from both material science and medical research. Here are just a few of the ways this is happening:
Predictive Modeling: Machine learning algorithms can predict the properties of new materials based on their chemical compositions and structures. This capability enables researchers to identify promising candidates for biocompatible materials without the need for extensive and time-consuming experimental testing (arXiv, 2016; Phys.org, 2020; ACS Publications, 2024; ScienceDaily, 2019; Nature, 2018).
Optimization of Material Properties: AI can optimize material properties such as strength, flexibility, and biocompatibility by learning from previous experiments and simulations. This iterative process refines materials to meet specific medical requirements, ensuring better performance and safety (Prezi, n.d.; Growth Garage, n.d.; FasterCapital, 2024).
Cross-Disciplinary Data Integration: AI facilitates the integration of diverse datasets from material science and medicine, uncovering patterns and correlations that might be missed by traditional methods. This holistic view accelerates the identification of materials that are not only biocompatible but also possess the desired mechanical and chemical properties for medical applications (ScienceDirect, 2024; ResearchGate, 2023).
Case Studies: AI-Driven Innovations in Biocompatible Materials
Case Study 1: Development of Titanium-Based Alloys for Implants
Titanium and its alloys are widely used in medical implants due to their excellent biocompatibility and mechanical properties. However, developing new titanium-based alloys with enhanced performance requires extensive experimentation. Researchers at a leading university (the university was not named in cited research papers) utilized machine learning algorithms to analyze data from thousands of alloy compositions and their properties. The AI model predicted several new titanium alloys with superior strength and corrosion resistance, which were subsequently validated through laboratory testing. These new alloys promise to improve the longevity and reliability of implants such as hip replacements and dental implants (ResearchGate, 2023; Frontiers in Materials, 2024; ACS Omega, 2022).
Case Study 2: Polymer Scaffolds for Tissue Engineering
Tissue engineering aims to regenerate damaged tissues using scaffolds made from biocompatible polymers. A research team employed AI to screen a vast library of polymer compositions and their interactions with biological tissues. The machine learning model identified a novel polymer blend that exhibited excellent cell adhesion, proliferation, and biodegradability. This new material was successfully used to create scaffolds for regenerating cartilage tissue, demonstrating the potential of AI in accelerating the development of advanced materials for regenerative medicine (Google Patents, n.d.; Journal of Biomedical Science, 2018; ScienceDirect, 2023).
Case Study 3: Biodegradable Stents for Cardiovascular Applications
Cardiovascular stents are critical in treating blocked arteries, but traditional metal stents pose risks such as inflammation and thrombosis. Researchers explored biodegradable polymers as an alternative, using AI to predict their degradation rates and mechanical performance. The AI-driven approach led to the discovery of a new polymer blend that degrades safely within the body while maintaining structural integrity during the healing process. Clinical trials have shown promising results, highlighting the potential for AI to revolutionize cardiovascular treatments (Harvard ADS, 2024; SpringerOpen, 2017; Academia.edu, n.d.).
Conclusion
The fusion of material science and medicine, powered by AI, is ushering in a new era of innovation in healthcare. By leveraging machine learning algorithms to analyze and predict material properties, researchers can rapidly develop biocompatible materials tailored for medical applications. The case studies presented demonstrate how AI-driven interdisciplinary research is transforming the landscape of medical implants, leading to safer, more effective, and longer-lasting solutions for patients.
As we continue to explore the synergies between these fields, the potential for AI to drive further advancements in biocompatible materials is limitless. The future of healthcare lies in the seamless integration of diverse scientific disciplines, with AI at the helm of this transformative journey.
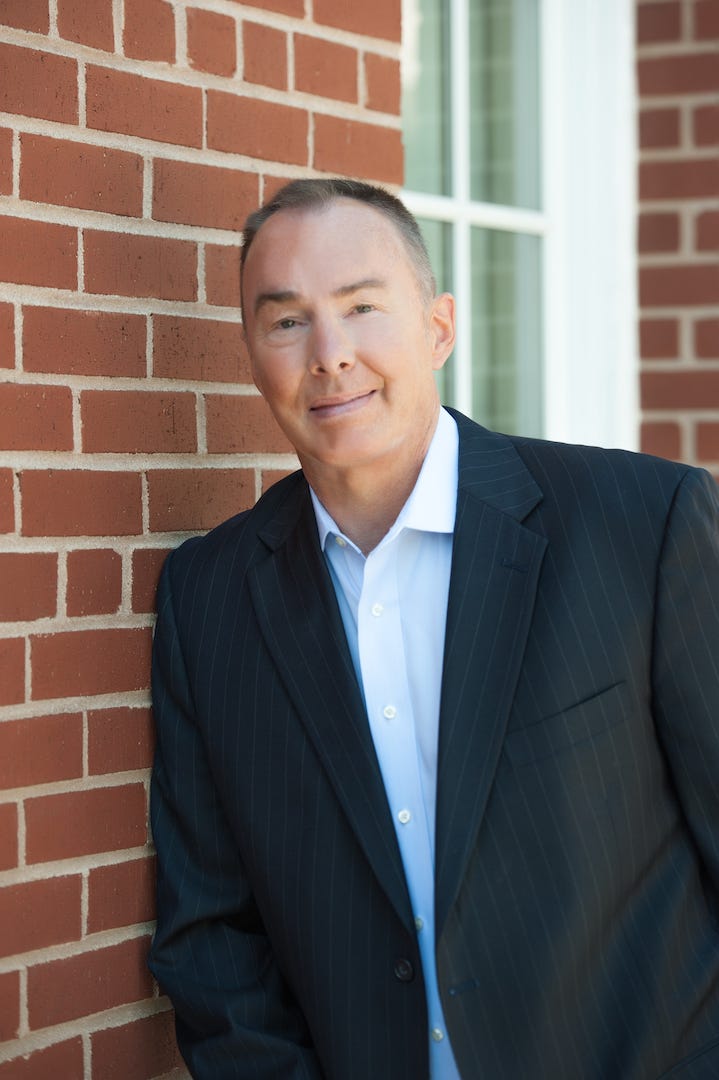
About the Author: David Ragland is a former senior technology executive and an adjunct professor of management. He serves as a partner at FuturePoint Digital, a research-based AI consultancy specializing in strategy, advisory & educational services, as well as custom AI application development. David earned his Doctorate in Business Administration from IE University in Madrid, Spain, and a Master of Science in Information and Telecommunications Systems from Johns Hopkins University. He also holds an undergraduate degree in Psychology from James Madison University and completed a certificate in Artificial Intelligence and Business Strategy at MIT. His research focuses on the intersection of artificial intelligence with organizational and societal dynamics.
References
ACS Omega. (2022, August 15). Effect of alloying elements on the compressive mechanical properties of titanium alloys. Retrieved from https://pubs.acs.org/doi/10.1021/acsomega.2c02096
ACS Publications. (2024, May 27). Editorial: Machine learning in materials science. Retrieved from https://pubs.acs.org/doi/10.1021/acs.jcim.4c00727
arXiv. (2016). A general-purpose machine learning framework for predicting properties of materials. Retrieved from https://arxiv.org/pdf/1606.09551
Britannica. (n.d.). Materials science. In Encyclopedia Britannica. Retrieved from https://www.britannica.com/technology/materials-science
FasterCapital. (2024, April 20). The future of material science entrepreneurship: Trends and opportunities to watch. Retrieved from https://fastercapital.com/content/The-Future-of-Material-Science-Entrepreneurship--Trends-and-Opportunities-to-Watch.html
Frontiers in Materials. (2024). A framework for computer-aided high performance titanium alloy design. Retrieved from https://www.frontiersin.org/journals/materials/articles/10.3389/fmats.2024.1364572/full
Google Patents. (n.d.). Novel biodegradable elastomeric scaffold for tissue engineering. Retrieved from https://patents.google.com/patent/US20120322155A1/en
Growth Garage. (n.d.). The impact of AI on polymer research. Retrieved from https://growthgarage.mcgc.com/ja/resources/the-impact-of-ai-on-polymer-research
Journal of Biomedical Science. (2018, December 20). Current and novel polymeric biomaterials for neural tissue engineering. Retrieved from https://jbiomedsci.biomedcentral.com/articles/10.1186/s12929-018-0491-8
MDPI. (2023, June 7). Biomaterials as implants in the orthopedic field for regenerative medicine. MDPI Polymers. Retrieved from https://www.mdpi.com/2073-4360/15/12/2601
Medical News Today. (2018, November 16). What is medicine? Definition, fields, and branches. Retrieved from https://www.medicalnewstoday.com/articles/323679
Nature. (2018, December 4). ElemNet: Deep learning the chemistry of materials from only elemental composition. Retrieved from https://www.nature.com/articles/s41598-018-35934-y
Phys.org. (2020, August 7). Algorithm predicts the compositions of new materials. Retrieved from https://phys.org/news/2020-08-algorithm-compositions-materials.html
Prezi. (n.d.). SDS - Final Draft by Yusuf Eldarieby. Retrieved from
ResearchGate. (2023, August 25). Unleashing the power of artificial intelligence in materials design. Retrieved from https://www.researchgate.net/publication/373535859_Unleashing_the_Power_of_Artificial_Intelligence_in_Materials_Design
ResearchGate. (2023, September 10). Predicting low-modulus biocompatible titanium alloys using machine learning. Retrieved from https://www.researchgate.net/publication/374156497_Predicting_Low-Modulus_Biocompatible_Titanium_Alloys_Using_Machine_Learning
ScienceDaily. (2019, May 15). Machine learning predicts mechanical properties of porous materials. Retrieved from https://www.sciencedaily.com/releases/2019/05/190515110337.htm
ScienceDirect. (2023). Conjugated polymer-based composite scaffolds for tissue engineering. Retrieved from https://www.sciencedirect.com/science/article/pii/S1110016823011328
ScienceDirect. (2024). Artificial intelligence for clinical prediction: Exploring key domains. Retrieved from https://www.sciencedirect.com/science/article/pii/S2666990024000156