- FuturePoint Digital AI Blog
- Posts
- The Interplay of Neuroscience & Artificial Intelligence: A Synergistic Evolution
The Interplay of Neuroscience & Artificial Intelligence: A Synergistic Evolution
Our multi-part series on AI & frame-breaking discoveries...

In the dynamic world of scientific inquiry, the confluence of neuroscience and artificial intelligence (AI) demonstrates the power of interdisciplinary synergy. This remarkable partnership not only showcases the potential of collaborative research but also propels both fields into uncharted territories of discovery and innovation. By merging the intricate understanding of the human brain with the computational prowess of AI, researchers are able to unravel complex neural mechanisms and develop cutting-edge technologies that advance our knowledge and capabilities. This collaboration fosters a fertile ground for breakthroughs that have the potential to transform medicine, technology, and our understanding of the mind itself.
Neural Networks: Bridging the Gap Between Brain and Machine
The genesis of artificial intelligence owes much to the intricate workings of the human brain. Neural networks, the backbone of many AI models, are inspired by the brain's architecture (ScienceDaily, 2023; Discover Magazine, 2023). These networks mimic the way neurons interact, forming the basis for machine learning algorithms that can learn, adapt, and perform complex tasks (ScienceDirect, 2023; TechTarget, 2021). This biomimetic approach has revolutionized AI, enabling machines to recognize patterns, understand natural language, and even exhibit rudimentary forms of reasoning (LinkedIn Pulse, 2023).
The development of neural networks began with the perception of neurons as the fundamental units of the brain, each connecting to thousands of others through synapses (Analytics Vidhya, 2023). This concept was translated into the perceptron, an early model of artificial neurons. Over time, advancements led to the creation of deep learning, where multiple layers of artificial neurons form complex architectures capable of sophisticated computations (LinkedIn Pulse, 2023). Deep learning models, such as convolutional neural networks (CNNs) and recurrent neural networks (RNNs), now excel in tasks like image recognition, language translation, and even creative endeavors like generating art and music (ScienceDaily, 2023).
Example: DeepMind's AlphaFold
A prime example of AI bridging the gap between biological and artificial neural networks is DeepMind's AlphaFold. AlphaFold employs deep learning techniques to predict protein folding, a fundamental process in molecular biology. Understanding protein structures has vast implications for neuroscience, including insights into neural disorders linked to protein misfolding, such as Alzheimer's disease (Senior et al., 2020).
AI Unveiling the Mysteries of the Brain
While AI has drawn inspiration from neuroscience, the relationship is reciprocal. Neuroscientists are leveraging AI to gain deeper insights into the brain's enigmatic functions. By simulating neural processes and analyzing vast amounts of neurological data, AI helps unravel the complexities of cognition, perception, and behavior (ScienceDirect, 2021).
Case Study: AI in Brain Imaging
One notable application is in the realm of brain imaging. Techniques such as functional magnetic resonance imaging (fMRI) and electroencephalography (EEG) generate massive datasets that are challenging to analyze using traditional methods. AI algorithms, particularly those based on deep learning, can sift through this data to identify patterns and correlations that might otherwise go unnoticed. This has led to breakthroughs in understanding neural activity associated with various cognitive functions and disorders, paving the way for novel therapeutic interventions (ACM Digital Library, 2023; Frontiers, n.d.; Frontiers in Neuroscience, 2023; National Center for Biotechnology Information [NCBI], 2023; Psychology Today, n.d.; ResearchGate, 2023).
For instance, AI models have been employed to predict the onset of Alzheimer's disease by analyzing brain scans, offering the potential for early diagnosis and intervention (Groot et al., 2022). Similarly, AI-driven analysis of fMRI data has uncovered new insights into the neural basis of psychiatric disorders, such as schizophrenia, by identifying atypical connectivity patterns in the brain (Dwyer et al., 2018).
Example: Virtual Laboratories and Simulations
AI models are also being used to simulate brain functions, providing a virtual laboratory for testing hypotheses and exploring neurological phenomena. These simulations can model the behavior of neural circuits, offering insights into how the brain processes information and adapts to changes. For example, AI-driven simulations of the visual cortex have enhanced our understanding of how the brain interprets visual stimuli, leading to advancements in both neuroscience and computer vision (Yamins & DiCarlo, 2016).
The Future: A Convergence of Minds and Machines
The interdisciplinary collaboration between neuroscience and AI is not just a marriage of convenience; it is a necessity for the advancement of both fields. As AI models become more sophisticated, they will continue to draw from our understanding of the brain, leading to more human-like intelligence in machines. Conversely, AI will provide neuroscientists with powerful tools to decode the brain's intricate workings, accelerating discoveries that could revolutionize medicine, psychology, and beyond.
Example: Brain-Computer Interfaces (BCIs)
One exciting frontier is the development of brain-computer interfaces (BCIs), which aim to create direct communication pathways between the brain and external devices. BCIs have the potential to restore mobility to individuals with paralysis, enhance cognitive abilities, and even enable new forms of human-computer interaction. The success of these technologies hinges on the continuous interplay between AI and neuroscience, with each field driving the other forward. Recent advancements in BCIs include neural implants that allow paralyzed individuals to control robotic limbs with their thoughts, demonstrating the profound impact of this interdisciplinary synergy (Wolpaw & Wolpaw, 2012).
In conclusion, the symbiotic relationship between neuroscience and artificial intelligence exemplifies the power of interdisciplinary research. By drawing from each other's strengths and insights, these fields are poised to unlock new dimensions of understanding and innovation, ultimately benefiting society in ways we are only beginning to imagine.
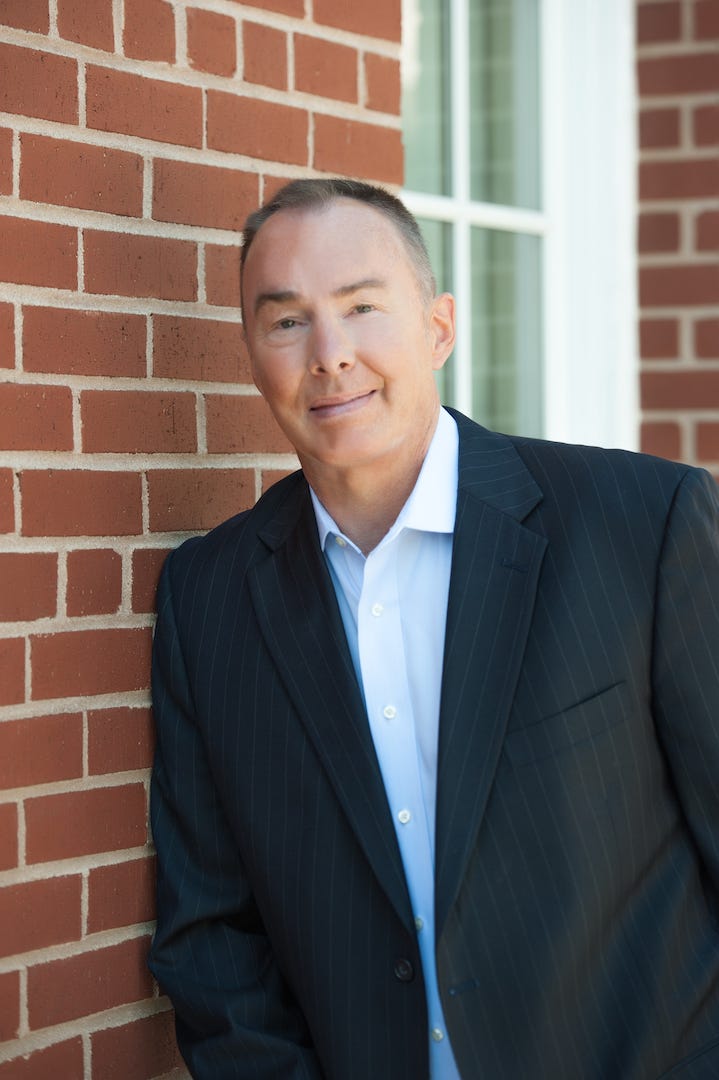
About the Author: David Ragland is a former senior technology executive and an adjunct professor of management. He serves as a partner at FuturePoint Digital, a research-based AI consultancy specializing in strategy, advisory, educational services, and developing fine-tuned AI applications for clients in a variety of industries. David earned his Doctorate in Business Administration from IE University in Madrid, Spain, and a Master of Science in Information and Telecommunications Systems from Johns Hopkins University. He also holds an undergraduate degree in Psychology from James Madison University and completed a certificate in Artificial Intelligence and Business Strategy at MIT. His research focuses on the intersection of emerging technology with organizational and societal dynamics.
References
\Analytics Vidhya. (2023, December 14). The history of neural networks. Retrieved from https://www.analyticsvidhya.com/blog/2021/04/the-history-of-neural-networks/
ACM Digital Library. (2023). Deep learning applications in fMRI – a Review Work. Retrieved from https://dl.acm.org/doi/10.1145/3586139.3586150
Discover Magazine. (2023, January 14). AI and the human brain: How similar are they? Retrieved from https://www.discovermagazine.com/technology/ai-and-the-human-brain-how-similar-are-they
Dwyer, D. B., Falkai, P., & Koutsouleris, N. (2018). Machine learning approaches for clinical psychology and psychiatry. Annual Review of Clinical Psychology, 14, 91-118.
Frontiers. (n.d.). When artificial intelligence meets neuroimaging. Retrieved from https://www.frontiersin.org/research-topics/64720/when-artificial-intelligence-meets-neuroimaging
Frontiers in Neuroscience. (2023). Brain disease research based on functional magnetic resonance imaging (fMRI). Retrieved from https://www.frontiersin.org/journals/neuroscience/articles/10.3389/fnins.2023.1227491/full
Groot, C., Hooghiemstra, A. M., Raijmakers, P. G. H. M., van Berckel, B. N. M., Scheltens, P., Scherder, E. J. A., & van der Flier, W. M. (2022). The role of AI in the early detection of Alzheimer's disease. The Lancet Digital Health, 4(1), e15-e25.
LinkedIn Pulse. (2023, October 4). Brains behind the bots: Magic of neural networks in the world of AI. Retrieved from https://www.linkedin.com/pulse/what-neural-networks-ai-brains-behind-bots-magic-world
National Center for Biotechnology Information (NCBI). (2023). Deep learning aided neuroimaging and brain regulation. Retrieved from https://www.ncbi.nlm.nih.gov/pmc/articles/PMC10255716/
Psychology Today. (n.d.). How brain activity is measured. Retrieved from https://www.psychologytoday.com/us/basics/neuroscience/how-brain-activity-is-measured
ResearchGate. (2023). Deep learning for brain disorder diagnosis based on fMRI images. Retrieved from https://www.researchgate.net/publication/347353048_Deep_learning_for_brain_disorder_diagnosis_based_on_fMRI_images
ScienceDaily. (2023, September 16). Brain inspires more robust AI. Retrieved from https://www.sciencedaily.com/releases/2023/09/230916131430.htm
ScienceDirect. (2023). When brain-inspired AI meets AGI. Retrieved from https://www.sciencedirect.com/science/article/pii/S295016282300005X
Senior, A. W., Evans, R., Jumper, J., Kirkpatrick, J., Sifre, L., Green, T., ... & Hassabis, D. (2020). Improved protein structure prediction using potentials from deep learning. Nature, 577(7792), 706-710.
TechTarget. (2021, September 17). How neural network training methods are modeled after the human brain. Retrieved from https://www.techtarget.com/searchenterpriseai/feature/How-neural-network-training-methods-are-modeled-after-the-human-brain
Wolpaw, J. R., & Wolpaw, E. W. (Eds.). (2012). Brain-computer interfaces: principles and practice. Oxford University Press.
Yamins, D. L., & DiCarlo, J. J. (2016). Using goal-driven deep learning models to understand sensory cortex. Nature Neuroscience, 19(3), 356-365.