- FuturePoint Digital AI Blog
- Posts
- Bioinformatics and Social Sciences: Leveraging AI to Bridge the Gap
Bioinformatics and Social Sciences: Leveraging AI to Bridge the Gap
Our multi-part series on AI & frame-breaking discoveries...

In recent years, the convergence of bioinformatics and social sciences has emerged as a promising interdisciplinary frontier. Bioinformatics, traditionally focused on the analysis of biological data, and social sciences, which examine human behavior and societal structures, may seem worlds apart. However, advancements in artificial intelligence (AI) and machine learning are enabling the integration of these fields, offering new opportunities to uncover insights that were previously out of reach. This blog explores how AI can bridge the gap between bioinformatics and social sciences by analyzing large datasets from both fields, with a particular focus on the potential to reveal correlations between genetic markers and social behaviors.
The Power of AI in Data Analysis
AI and machine learning algorithms excel at processing vast amounts of data, identifying patterns, and making predictions. In bioinformatics, these tools are already used to analyze genomic sequences, protein structures, and other biological datasets. Similarly, in social sciences, AI is employed to study social networks, behavior patterns, and demographic data. The true potential lies in combining these datasets to gain a holistic understanding of human behavior from both a biological and social perspective (ITRex Group, 2023; Mayo Clinic Research, 2023; Omics Tutorials, 2023; Towards Data Science, 2020).
Identifying Correlations Between Genetic Markers and Social Behaviors
One of the most intriguing applications of AI in this interdisciplinary effort is the identification of correlations between genetic markers and social behaviors. Behavioral genetics is a field that explores the role of genetics in shaping behavior. By leveraging AI, researchers can analyze genetic data alongside social science datasets to identify patterns that might explain how certain genetic markers influence behaviors such as aggression, empathy, risk-taking, and social interaction (Wurth, 2022; Zwir, 2019; Wikipedia, 2024).
From a practical perspective, this interdisciplinary approach holds significant potential for advancing our understanding of human behavior. Machine learning algorithms can be trained to find associations between specific genetic variations and behavioral traits by analyzing large-scale genomic data and comprehensive social behavior surveys. This approach can help identify genetic predispositions to certain behaviors and how these predispositions interact with environmental factors (News-Medical, 2024; Frontiers, 2019).
By integrating AI and machine learning with behavioral genetics, researchers can uncover the genetic underpinnings of social behaviors and their interaction with the environment. This holistic approach has the potential to transform personalized medicine, inform social policies, and foster interdisciplinary research, ultimately leading to a deeper understanding of human behavior and improved societal outcomes (News-Medical, 2019; Liu, 2023; PNAS, 2023; Neuroscience News, 2019).
Case Studies and Empirical Evidence
Several studies have already demonstrated the potential of AI in bridging bioinformatics and social sciences. One notable study that demonstrates the potential of AI in bridging bioinformatics and social sciences is the research conducted by the Frontiers in Neuroscience. This study used machine learning models to analyze genetic data and psychological profiles to understand the genetic basis of anxiety and depression. By examining both genetic markers and social factors, the researchers were able to identify new genetic variants associated with these conditions, offering deeper insights into their underlying mechanisms (Frontiers in Neuroscience, 2022).
Another study focused on the genetic basis of educational attainment. The research, published in Nature Genetics, demonstrates the integration of AI to uncover genetic markers related to educational attainment. This study conducted a genome-wide association study (GWAS) of educational attainment involving approximately 3 million individuals. The researchers identified 3,952 genetic variants associated with educational attainment by combining genetic data with extensive educational and socioeconomic data, providing new insights into the complex interplay between genetics and social environments (Nature Genetics, 2022).
Future Directions and Implications
The integration of bioinformatics and social sciences through AI holds significant promise for various fields, including medicine, psychology, sociology, and public health. By understanding the genetic underpinnings of social behaviors, we can develop more targeted interventions and personalized treatments for mental health disorders, improve educational strategies, and create more effective public policies.
Moreover, this interdisciplinary approach can foster a more comprehensive understanding of human behavior, considering both biological and social dimensions. It encourages collaboration between biologists, social scientists, data scientists, and AI experts, leading to innovative research methodologies and groundbreaking discoveries.
Conclusion
AI is revolutionizing the way we approach the study of human behavior by bridging the gap between bioinformatics and social sciences. By analyzing large datasets from both fields, machine learning algorithms can identify correlations between genetic markers and social behaviors, providing new insights into behavioral genetics. The potential applications are vast and hold the promise of advancing our understanding of human behavior, improving mental health treatment, and informing public policy. As we continue to explore this interdisciplinary frontier, the synergy between bioinformatics and social sciences, powered by AI, will undoubtedly lead to exciting new discoveries and innovations.
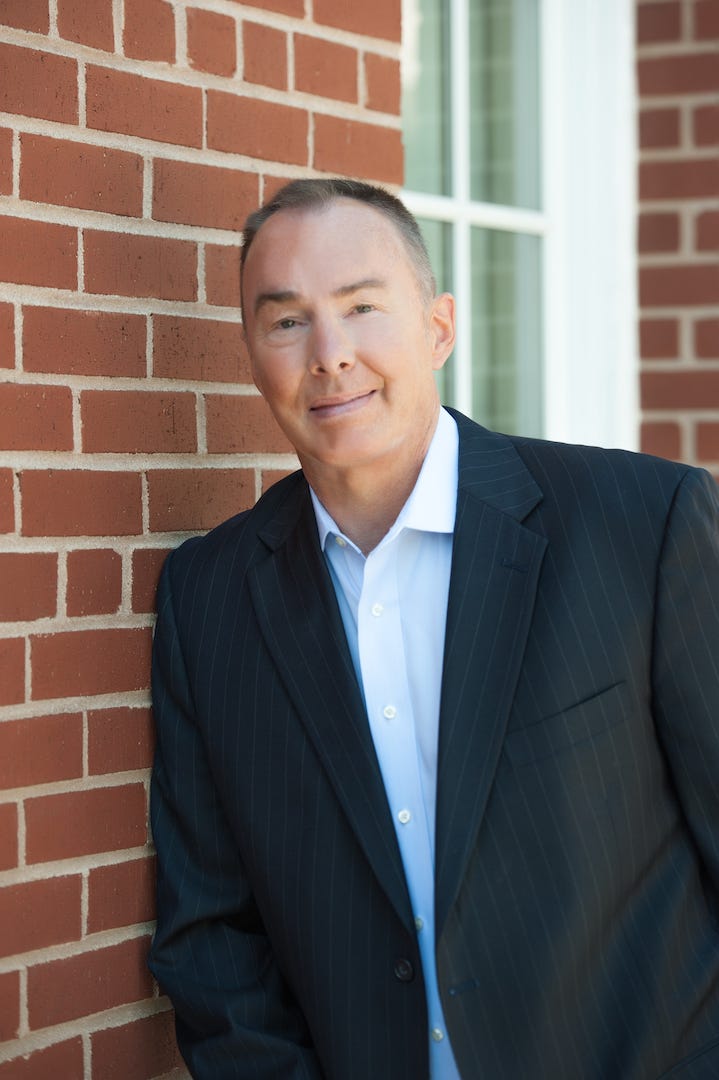
About the Author: David Ragland is a former senior technology executive and an adjunct professor of management. He serves as a partner at FuturePoint Digital, a research-based technology consultancy specializing in strategy, advisory, and educational services for global clients. David earned his Doctorate in Business Administration from IE University in Madrid, Spain, and a Master of Science in Information and Telecommunications Systems from Johns Hopkins University. He also holds an undergraduate degree in Psychology from James Madison University and completed a certificate in Artificial Intelligence and Business Strategy at MIT. His research focuses on the intersection of emerging technology with organizational and societal dynamics
References
Frontiers. (2019, June 26). The genome-wide study of human social behavior and genetics. Retrieved from https://www.frontiersin.org/journals/sociology/articles/10.3389/fsoc.2019.00053/full
Frontiers in Neuroscience. (2022, August 8). A machine learning model for predicting patients with major depressive disorder: A study based on transcriptomic data. Retrieved from https://www.frontiersin.org/journals/neuroscience/articles/10.3389/fnins.2022.949609/full
ITRex Group. (2023, February 7). AI and machine learning in bioinformatics. Retrieved from https://itrexgroup.com/blog/ai-and-machine-learning-in-bioinformatics/
Liu, X. (2023, June 30). New AI tool can help track and identify complex fruit fly behavior. Retrieved from https://www.news-medical.net/news/20230630/New-AI-tool-can-help-track-and-identify-complex-fruit-fly-behavior.aspx
Mayo Clinic Research. (2023). Artificial Intelligence and Informatics. Retrieved from https://www.mayo.edu/research/departments-divisions/artificial-intelligence-informatics/overview
Nature Genetics. (2022, March 31). Polygenic prediction of educational attainment within and between populations. Nature Genetics. Retrieved from https://www.nature.com/articles/s41588-022-01016-z
Neuroscience News. (2019, August 13). Machine learning decodes genetic influence over behavior. Retrieved from https://neurosciencenews.com/machine-learning-genetics-behavior-14705/
News-Medical. (2019, August 15). Machine learning reveals links between genetic factors and behavior. Retrieved from https://www.news-medical.net/news/20190815/Machine-learning-reveals-links-between-genetic-factors-and-behavior.aspx
News-Medical. (2024, March 4). AI-powered transfer learning approach predicts gene perturbations that transform cell behavior. Retrieved from https://www.news-medical.net/news/20240304/AI-powered-transfer-learning-approach-predicts-gene-perturbations-that-transform-cell-behavior.aspx
Omics Tutorials. (2023, May 17). Role of artificial intelligence and machine learning in bioinformatics. Retrieved from https://omicstutorials.com/role-of-artificial-intelligence-and-machine-learning-in-bioinformatics/
PNAS. (2023, April 17). What can machine learning teach us about habit formation. Retrieved from https://www.pnas.org/doi/10.1073/pnas.2216115120
Towards Data Science. (2020, March 31). Bioinformatics: How AI can contribute to the study of life. Retrieved from https://towardsdatascience.com/bioinformatics-how-ai-can-contribute-to-the-study-of-life-7a67f3d62a9f
Wikipedia. (2024). Behavioral genetics. Retrieved from https://en.wikipedia.org/wiki/Behavioural_genetics
Wurth, A. (2022). Behavior and genetic aspects of boldness and aggression in urban wildlife. Retrieved from https://senr.osu.edu/sites/senr/files/imce/files/TWEL/ThesisDissertations/Wurth%2C%20Ashley-Dissertation.pdf
Zwir, I. (2019, November 20). AI helps identify gene, environment networks that shape personality. Retrieved from https://medicine.wustl.edu/news/gene-environment-interactions-help-shape-personality/