- FuturePoint Digital AI Blog
- Posts
- Astrophysics and Data Science: AI's Role in Propelling Interdisciplinary Discoveries
Astrophysics and Data Science: AI's Role in Propelling Interdisciplinary Discoveries
Our multi-part series on AI & frame-breaking discoveries...

Astrophysics is the branch of astronomy that deals with the physical nature of celestial objects and phenomena. It involves the study of the universe's structure, origins, and the fundamental laws governing these entities. Data Science, on the other hand, encompasses methods for collecting, analyzing, and interpreting vast amounts of data. When combined, these fields leverage the power of AI to unravel the mysteries of the cosmos in unprecedented ways.
The Role of AI in Astrophysics
AI, particularly machine learning (ML), has become an indispensable tool in astrophysics due to its capability to process and analyze large datasets rapidly and accurately. Astronomical data, gathered from telescopes and space missions, can be overwhelmingly extensive and complex. Machine learning algorithms are adept at identifying patterns and making predictions, which are critical for discovering new celestial bodies, understanding cosmic phenomena, and even detecting signals of extraterrestrial life.
Machine learning has revolutionized the field of astrophysics by automating the classification of astronomical objects, a task that traditionally required significant human effort. For instance, convolutional neural networks (CNNs) have been successfully employed to classify galaxies based on their morphologies (Huertas-Company et al., 2018). This automation not only accelerates the research process but also improves the accuracy of classifications, thereby facilitating a deeper understanding of the universe's structure. Moreover, ML algorithms have been pivotal in analyzing data from gravitational wave detectors, enabling the detection of these elusive phenomena with unprecedented precision (Abbott et al., 2016).
In addition to classification and detection, machine learning enhances our ability to predict and simulate cosmic events. For example, generative adversarial networks (GANs) are used to create realistic simulations of the universe, which help astrophysicists to test theories and models against synthetic data (Ravanbakhsh et al., 2017). Furthermore, ML algorithms have proven effective in predicting the behavior of complex systems, such as the dynamics of star formation and the evolution of galaxies over time (Cameron et al., 2019). These predictive capabilities are essential for advancing our knowledge of astrophysical processes and for planning future observational campaigns.
For instance, AI has been utilized to classify galaxies in astronomical surveys, detect exoplanets in light curves from space telescopes, and predict solar activity. A notable example is the application of convolutional neural networks (CNNs) to analyze images from the Hubble Space Telescope, enabling the discovery of previously unnoticed celestial objects (Zhu et al., 2019).
The Role of AI in Data Science
In data science, AI aids in extracting meaningful insights from vast datasets across various domains. Machine learning models can handle large volumes of data, identify trends, and make predictions that are not immediately obvious through traditional statistical methods. These capabilities are crucial in fields like healthcare, finance, and social sciences, where data-driven decision-making is paramount.
In healthcare, machine learning algorithms are employed to predict disease outbreaks, personalize treatment plans, and even diagnose conditions from medical imaging. For example, deep learning models have demonstrated remarkable accuracy in detecting diabetic retinopathy from retinal images, outperforming human experts (Gulshan et al., 2016). In the financial sector, AI-driven predictive analytics help in risk management, fraud detection, and algorithmic trading. Studies have shown that ML models can predict stock market trends and credit risks with higher accuracy compared to traditional approaches (Feng et al., 2019). In the social sciences, AI is used to analyze social media data to understand public sentiment, predict election outcomes, and study human behavior on a large scale. Machine learning models have been particularly effective in analyzing large-scale text data, identifying patterns and trends that inform policy and business strategies (Batrinca & Treleaven, 2015).
The integration of AI in data science thus enhances the capacity to process and interpret complex datasets, driving innovation and improving decision-making processes across various fields. By uncovering hidden patterns and making accurate predictions, AI not only augments traditional analytical methods but also opens new avenues for research and application.
AI Propelling Interdisciplinary Discoveries
The fusion of astrophysics and data science through AI-driven techniques is yielding remarkable interdisciplinary discoveries. AI's ability to process and interpret astronomical data has led to breakthroughs in understanding the universe's structure and origins.
Case Study: Exoplanet Detection
One example of AI propelling interdisciplinary research is the use of machine learning in exoplanet detection. Traditional methods of detecting exoplanets, such as the transit method, require meticulous examination of light curves to identify potential planets. This process can be time-consuming and prone to human error. AI, specifically deep learning models, can analyze light curves from space telescopes like Kepler with greater speed and accuracy (Shallue & Vanderburg, 2018). These models have been trained to recognize the subtle dips in brightness that indicate a planet passing in front of its host star, significantly reducing the time required to identify exoplanet candidates and increasing the detection rate (Pearson et al., 2018).
A study by Shallue and Vanderburg (2018) demonstrated that a deep learning algorithm could effectively identify exoplanets by analyzing light curves. This AI-driven approach led to the discovery of several new exoplanets that were previously overlooked by human researchers.
Case Study: Galaxy Classification
Another example is the use of AI for classifying galaxies. The Sloan Digital Sky Survey (SDSS) has collected millions of galaxy images, which are essential for studying the universe's structure and evolution. Traditional classification methods involve manual categorization, which is not feasible given the sheer volume of data.
Using convolutional neural networks, researchers have developed models that can classify galaxies with high accuracy. A study by Dieleman et al. (2015) showed that a CNN could classify galaxy morphologies based on SDSS images, significantly accelerating the data analysis process and enabling researchers to focus on interpreting results and making new discoveries.
Conclusion
AI's integration into astrophysics and data science is revolutionizing how we explore and understand the universe. By leveraging machine learning techniques, researchers can process astronomical data more efficiently, leading to groundbreaking discoveries about the cosmos. These interdisciplinary advancements highlight the transformative potential of AI in scientific research.
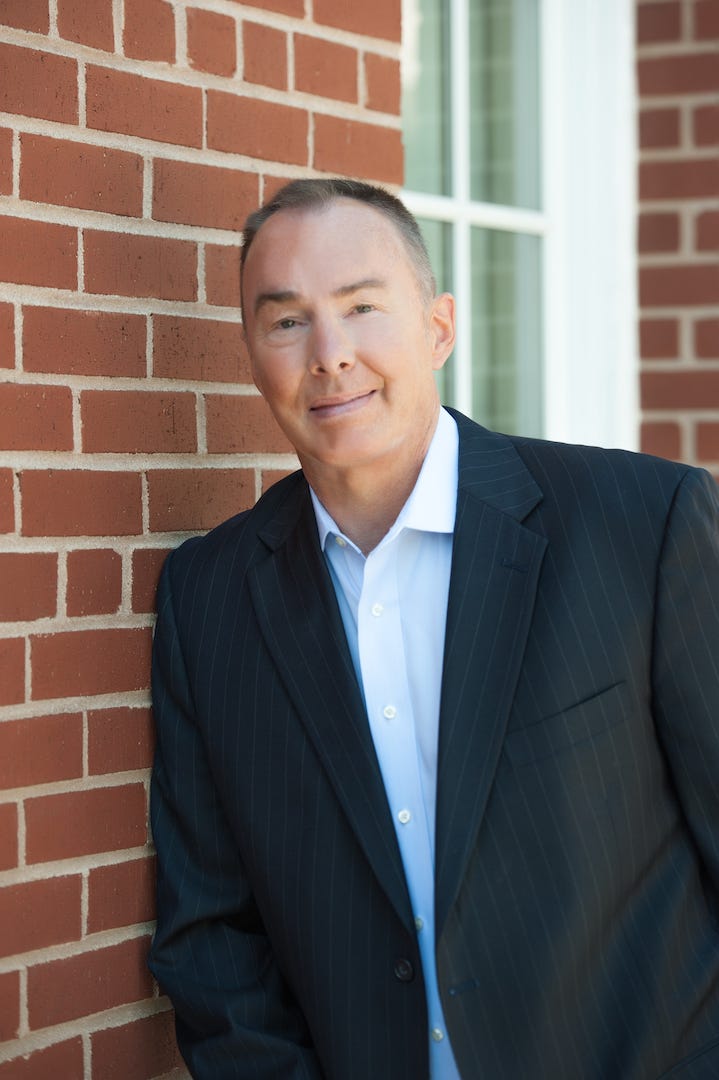
About the Author: David Ragland is a former senior technology executive and an adjunct professor of management. He serves as a partner at FuturePoint Digital, a research-based AI consultancy specializing in strategy, advisory & educational services, as well as custom AI application development. David earned his Doctorate in Business Administration from IE University in Madrid, Spain, and a Master of Science in Information and Telecommunications Systems from Johns Hopkins University. He also holds an undergraduate degree in Psychology from James Madison University and completed a certificate in Artificial Intelligence and Business Strategy at MIT. His research focuses on the intersection of artificial intelligence with organizational and societal dynamics.
References
Abbott, B. P., Abbott, R., Abbott, T. D., Abernathy, M. R., Acernese, F., Ackley, K., ... & Zweizig, J. (2016). Observation of gravitational waves from a binary black hole merger. Physical Review Letters, 116(6), 061102.
Batrinca, B., & Treleaven, P. C. (2015). Social media analytics: A survey of techniques, tools and platforms. AI & Society, 30(1), 89-116.
Cameron, E., Carney, M., Brinckmann, T., & Green, S. (2019). AstroML: Machine learning and data mining for astronomy. arXiv preprint arXiv:1901.02443.
Dieleman, S., Willett, K. W., & Dambre, J. (2015). Rotation-invariant convolutional neural networks for galaxy morphology prediction. Monthly Notices of the Royal Astronomical Society, 450(2), 1441-1459. https://doi.org/10.1093/mnras/stv632
Feng, F., He, X., Wang, X., Luo, C., & Chua, T. S. (2019). Temporal relational ranking for stock prediction. ACM Transactions on Information Systems (TOIS), 37(2), 1-30.
Gulshan, V., Peng, L., Coram, M., Stumpe, M. C., Wu, D., Narayanaswamy, A., ... & Webster, D. R. (2016). Development and validation of a deep learning algorithm for detection of diabetic retinopathy in retinal fundus photographs. JAMA, 316(22), 2402-2410.
Huertas-Company, M., Bernardi, M., Tuccillo, D., Fischer, J. L., Margalef-Bentabol, B., Domínguez-Sánchez, H., ... & Xu, C. K. (2018). A catalogue of automatic morphological classifications for 670,000 galaxies from the Sloan Digital Sky Survey. Monthly Notices of the Royal Astronomical Society, 475(4), 4648-4669.
Pearson, K. A., Palafox, L., & Griffith, C. A. (2018). Searching for exoplanets using artificial intelligence. Monthly Notices of the Royal Astronomical Society, 474(1), 478-491.
Ravanbakhsh, S., Oliva, J. B., Fromenteau, S., Price, L. C., Ho, S., Schneider, J., & Poczos, B. (2017). Estimating cosmological parameters from the dark matter distribution. In Proceedings of the 31st International Conference on Neural Information Processing Systems (pp. 2310-2318).
Shallue, C. J., & Vanderburg, A. (2018). Identifying exoplanets with deep learning: A five-planet resonant chain around Kepler-80 and an eighth planet around Kepler-90. The Astronomical Journal, 155(2), 94. https://doi.org/10.3847/1538-3881/aa9e09
Zhu, X., Zhang, Z., & Wang, S. (2019). Deep learning for galaxy cluster studies. Nature Astronomy, 3, 764–769. https://doi.org/10.1038/s41550-019-0781-
#ArtificialIntelligence #MachineLearning #DeepLearning #AI #DataScience #BigData #Robotics #NLP (Natural Language Processing) #ComputerVision #AIResearch #TechTrends #AIethics #AIinnovation #FutureOfWork #Automation #SmartTechnology #IoT #DigitalTransformation #AIApplications #AIInBusiness