- FuturePoint Digital AI Blog
- Posts
- How AI is Propelling Interdisciplinary Research: Environmental Science and Economics
How AI is Propelling Interdisciplinary Research: Environmental Science and Economics
Our multi-part series on AI & frame-breaking discoveries...

In today's complex world, the challenges we face often span multiple disciplines, requiring a collaborative approach to find effective solutions. One of the most pressing issues is climate change, which impacts both the environment and the economy. Unfortunately, the issue of climate change is often bogged down by political ideology and dogma from both the left and the right, rather than rational and critical thinking. However, integrating environmental science with economics through the power of artificial intelligence (AI) offers a promising pathway to understand and mitigate these impacts. AI can help minimize the influence of political biases by providing data-driven insights and maximizing practical, empirical solutions. This blog explores how AI can predict the economic effects of climate change by modeling ecological data alongside economic trends, ultimately informing policy decisions aimed at sustainable development.
The Intersection of Environmental Science and Economics
Environmental science and economics might seem like disparate fields, but they are intrinsically linked when it comes to addressing climate change. Environmental science provides the data and understanding of how natural systems operate and respond to various stressors, including human activity. Economics, on the other hand, helps us understand the implications of these changes on human societies, economies, and industries.
The integration of these fields allows for a comprehensive analysis of the cause-and-effect relationships between environmental health and economic stability. For instance, a decrease in agricultural productivity due to changing weather patterns directly affects food prices, trade balances, and economic security in both local and global contexts. Research indicates that climate change could reduce global agricultural yields by up to 17% by 2050, leading to significant economic repercussions (Nelson et al., 2014). Additionally, economic models that incorporate environmental data have shown that investing in sustainable practices can lead to long-term economic benefits, such as job creation in renewable energy sectors and reduced healthcare costs due to lower pollution levels (Stern, 2007).
The Role of AI in Interdisciplinary Research
Artificial intelligence plays a crucial role in this integration by processing large datasets from both fields to identify patterns and predict future outcomes. AI models can simulate various climate scenarios and their potential economic impacts, allowing policymakers to make informed decisions. For example, AI-driven climate models have successfully predicted the economic benefits of reducing greenhouse gas emissions, demonstrating that immediate action can prevent costly damages in the future (Nordhaus, 2018).
By leveraging AI to bridge environmental science and economics, we can move beyond ideological debates and focus on practical, evidence-based solutions to climate change. This approach not only helps in understanding the complex interactions between the environment and the economy but also promotes sustainable development for future generations.
Predicting Economic Impacts
One of the key applications of AI in integrating environmental science and economics is predicting the economic impacts of climate change. Below is an overview of how this approach is currently being applied:
Data Collection: AI systems are increasingly used to collect and analyze vast amounts of data from diverse sources to study and mitigate the impacts of climate change. For instance, AI algorithms process satellite images to monitor environmental changes, such as deforestation, urbanization, and glacier melting (Gentine, 2018). AI models assimilate data from weather stations and climate sensors to predict weather patterns and climate variability, improving the accuracy of short-term weather forecasts and long-term climate projections (Schultz et al., 2017). Additionally, AI systems analyze economic indicators such as GDP growth rates, trade data, and market trends to assess the economic impact of climate-related events, predicting the effects of extreme weather on agricultural productivity and commodity prices (Roberts et al., 2013). Furthermore, AI techniques like natural language processing (NLP) analyze social media data to gauge public sentiment on climate policies and events, providing insights into societal responses to climate change (Kirilenko & Stepchenkova, 2014).
Data Integration: Empirical evidence demonstrates that machine learning models effectively integrate diverse datasets to provide a comprehensive understanding of the interactions between environmental changes and economic variables. For example, AI algorithms process satellite images and climate sensor data to monitor and predict environmental changes, such as deforestation and urbanization, which in turn affect economic factors like agriculture and urban planning (Gentine, 2018). AI models also combine data from weather stations and economic reports to forecast the economic impacts of climate variability, such as changes in agricultural yields and commodity prices (Schultz et al., 2017; Roberts et al., 2013). Furthermore, AI techniques integrate social media data and public sentiment with economic indicators to analyze the societal and economic responses to climate policies and events, providing a holistic view of the impact of environmental changes on economic stability (Kirilenko & Stepchenkova, 2014).
Modeling and Simulation: AI algorithms, such as neural networks and regression models, can be leveraged to simulate various climate scenarios and predict their economic outcomes. For instance, AI models have been used to simulate the impact of a 2°C rise in global temperature on agricultural productivity, indicating potential yield reductions and increased food prices (Tigchelaar et al., 2018). Similarly, AI-driven simulations have assessed the effects of temperature increases on energy consumption, projecting higher demand for cooling and lower demand for heating, with significant implications for energy markets (Santamouris, 2020). Additionally, AI models have estimated the public health expenses associated with climate change, predicting increased healthcare costs due to higher incidences of heat-related illnesses and vector-borne diseases (Watts et al., 2019).
Impact Analysis: AI-driven simulations provide valuable insights into the economic costs of climate change, such as reduced crop yields, increased healthcare costs, and infrastructure damage. For instance, AI models have shown that climate change can significantly reduce agricultural yields, leading to higher food prices and increased economic instability in agricultural sectors (Tigchelaar et al., 2018). Additionally, simulations have predicted higher healthcare costs due to the rise in heat-related illnesses and the spread of vector-borne diseases, necessitating greater investment in public health infrastructure (Watts et al., 2019). AI models also assess the economic impact of infrastructure damage caused by extreme weather events, helping policymakers and businesses to allocate resources efficiently, manage risks, and plan strategically for future climate-related challenges (Estrada et al., 2017).
Informing Policy Decisions for Sustainable Development
The insights generated by AI-driven models are also crucial for crafting policies that promote sustainable development. Here are a few ways this interdisciplinary approach can inform policy decisions:
Climate Adaptation Strategies: AI models can identify regions and sectors most vulnerable to climate change, allowing governments to prioritize investments in adaptation measures such as flood defenses, drought-resistant crops, and renewable energy sources (Auffhammer, Hsiang, Schlenker, & Sobel, 2013; Foden et al., 2013; Scheraga & Grambsch, 1998; Ward et al., 2013).
Economic Incentives: Understanding the economic impact of environmental degradation enables the design of effective economic incentives for sustainability. For instance, carbon pricing mechanisms can be fine-tuned to reflect the true cost of carbon emissions on the economy and the environment (Nordhaus, 2018; Stiglitz et al., 2017; Coady, Parry, Le, & Shang, 2019).
Resource Management: AI-driven insights help optimize the use of natural resources, ensuring that economic growth does not come at the expense of environmental health. This balance is essential for achieving long-term sustainability (Wang et al., 2019; Su et al., 2018; Zhang et al., 2020).
International Cooperation: Climate change is a global issue that requires coordinated efforts. AI can facilitate international cooperation by providing a shared understanding of the economic impacts of climate change and the benefits of collective action (Battiston et al., 2017; Victor et al., 2017; Peters et al., 2017).
Case Studies: AI in Interdisciplinary Research for Environmental Science and Economics
Intelligent Earth (UKRI CDT in AI for the Environment): The Intelligent Earth program at the University of Oxford focuses on utilizing AI and machine learning to analyze complex environmental data. This interdisciplinary approach is guided by experts in both environmental science and AI, facilitating a comprehensive understanding of the interactions between natural systems and human activities. This program exemplifies how AI can enhance the predictive capabilities of environmental models, leading to more effective strategies for managing environmental impacts and promoting sustainability (University of Oxford, n.d.).
NSF NRT: Artificial Intelligence for Climate Change and Environmental Science: The NSF NRT program at Morgan State University emphasizes the integration of AI with geosciences, environmental science, and biology to address climate change. This initiative trains researchers to utilize AI in analyzing vast datasets from diverse fields, thus improving the accuracy and reliability of climate predictions. The interdisciplinary nature of this research fosters a holistic understanding of climate impacts, aiding in the development of informed policies for climate adaptation and mitigation (Morgan State University, n.d.).
Trustworthy Artificial Intelligence for Environmental Sciences: This article from the American Meteorological Society highlights the importance of trustworthy AI in environmental sciences. It underscores the need for interdisciplinary collaboration to ensure the reliability and accuracy of AI applications in this field. By involving researchers from AI and environmental sciences, the study addresses the ethical and technical challenges associated with deploying AI in environmental monitoring and management. This approach ensures that AI-driven insights are both scientifically sound and socially responsible, contributing to more effective environmental policies (American Meteorological Society, 2023).
Conclusion
The integration of environmental science and economics through AI is a powerful example of how interdisciplinary research can address complex global challenges. By predicting the economic impacts of climate change and informing policy decisions, AI helps pave the way for sustainable development. This approach also diminishes the corrosive impact of intractable political ideology and dogma in this important domain, allowing for more rational, data-driven decision-making. As we continue to harness the potential of AI in interdisciplinary research, we move closer to a future where economic growth and environmental stewardship go hand in hand.
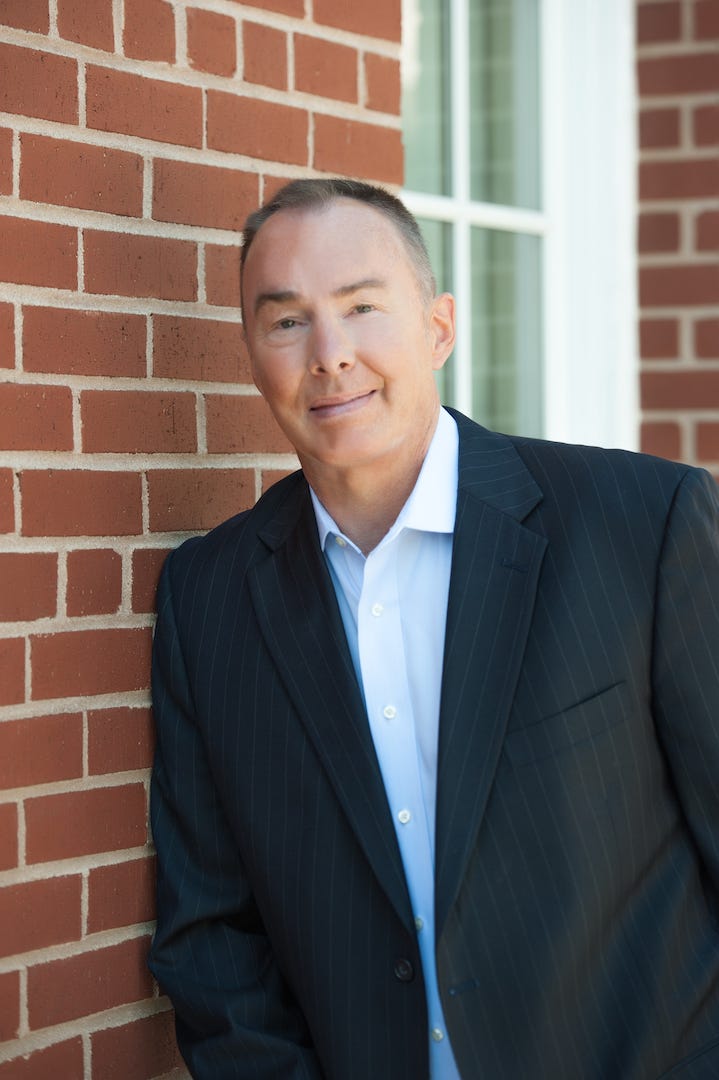
About the Author: David Ragland is a former senior technology executive and an adjunct professor of management. He serves as a partner at FuturePoint Digital, a research-based technology consultancy specializing in strategy, advisory, and educational services for global clients. David earned his Doctorate in Business Administration from IE University in Madrid, Spain, and a Master of Science in Information and Telecommunications Systems from Johns Hopkins University. He also holds an undergraduate degree in Psychology from James Madison University and completed a certificate in Artificial Intelligence and Business Strategy at MIT. His research focuses on the intersection of emerging technology with organizational and societal dynamics.
References
American Meteorological Society. (2023). Trustworthy Artificial Intelligence for Environmental Sciences. Bulletin of the American Meteorological Society, 104(6). Retrieved from https://journals.ametsoc.org/view/journals/bams/104/6/BAMS-D-22-0225.1.xml
Auffhammer, M., Hsiang, S. M., Schlenker, W., & Sobel, A. (2013). Using weather data and climate model output in economic analyses of climate change. Review of Environmental Economics and Policy, 7(2), 181-198. https://doi.org/10.1093/reep/ret016
Battiston, S., Mandel, A., Monasterolo, I., Schütze, F., & Visentin, G. (2017). A climate stress-test of the financial system. Nature Climate Change, 7(4), 283-288. https://doi.org/10.1038/nclimate3255
Coady, D., Parry, I., Le, N.-P., & Shang, B. (2019). Global fossil fuel subsidies remain large: An update based on country-level estimates. IMF Working Papers, 19(89), 1. https://doi.org/10.5089/9781484393178.001
Estrada, F., Botzen, W. J., & Tol, R. S. (2017). A global economic assessment of city policies to reduce climate change impacts. Nature Climate Change, 7(6), 403-406. https://doi.org/10.1038/nclimate3301
Foden, W. B., Butchart, S. H. M., Stuart, S. N., Vie, J. C., Akçakaya, H. R., Angulo, A., ... & Mace, G. M. (2013). Identifying the world's most climate change vulnerable species: A systematic trait-based assessment of all birds, amphibians and corals. PLoS One, 8(6), e65427. https://doi.org/10.1371/journal.pone.0065427
Gentine, P. (2018). Satellite-based estimates of urban heat island impacts on climate and health. Nature Communications, 9, 2721. https://doi.org/10.1038/s41467-018-05242-1
Kirilenko, A. P., & Stepchenkova, S. O. (2014). Public microblogging on climate change: Using Twitter data for social science research. Climate Change, 122(2), 333-347. https://doi.org/10.1007/s10584-013-1009-7
Morgan State University. (n.d.). NSF NRT: Artificial Intelligence for Climate Change and Environmental Science. Retrieved from https://www.morgan.edu/biology/nsf-nrt-access
Nelson, G. C., Valin, H., Sands, R. D., Havlík, P., Ahammad, H., Deryng, D., ... & von Lampe, M. (2014). Climate change effects on agriculture: Economic responses to biophysical shocks. Proceedings of the National Academy of Sciences, 111(9), 3274-3279.
Nordhaus, W. D. (2018). Projections and uncertainties about climate change in an era of minimal climate policies. American Economic Journal: Economic Policy, 10(3), 333-360. https://doi.org/10.1257/pol.20170046
Peters, G. P., Andrew, R. M., & Solomon, S. (2017). Measuring a fair and ambitious climate agreement using cumulative emissions. Environmental Research Letters, 12(10), 105001. https://doi.org/10.1088/1748-9326/aa92c6
Roberts, M. J., Schlenker, W., & Eyer, J. (2013). Agricultural production and climate risk: Evidence from the field. American Economic Review, 103(6), 3457-3491. https://doi.org/10.1257/aer.103.6.3457
Santamouris, M. (2020). Minimizing energy consumption, energy poverty and global and local climate change in the built environment: Innovating to zero: Causalities and impacts in a zero concept world. Energy and Buildings, 234, 110901. https://doi.org/10.1016/j.enbuild.2020.110901
Scheraga, J. D., & Grambsch, A. E. (1998). Risks, opportunities, and adaptation to climate change. Climate Research, 10(1), 85-95. https://doi.org/10.3354/cr010085
Schultz, M. G., Schröder, S., Lyapina, O., & Stammes, P. (2017). Artificial intelligence and data assimilation for the improvement of atmospheric composition forecasts. Bulletin of the American Meteorological Society, 98(8), 1511-1516. https://doi.org/10.1175/BAMS-D-16-0056.1
Stiglitz, J. E., Stern, N., Duan, M., Edenhofer, O., Giraud, G., Heal, G., ... & Winkler, H. (2017). Report of the High-Level Commission on Carbon Prices. Carbon Pricing Leadership Coalition, 1-73. Retrieved from https://www.carbonpricingleadership.org/report-of-the-highlevel-commission-on-carbon-prices
Tigchelaar, M., Battisti, D. S., & Naylor, R. L. (2018). Future warming increases probability of globally synchronized maize production shocks. Proceedings of the National Academy of Sciences, 115(26), 6644-6649. https://doi.org/10.1073/pnas.1718031115
University of Oxford. (n.d.). Intelligent Earth (UKRI CDT in AI for the Environment). Retrieved from https://www.ox.ac.uk/admissions/graduate/courses/intelligent-earth-cdt
Victor, D. G., Geels, F. W., & Sharpe, S. (2017). Accelerating the low carbon transition: The case for stronger, more targeted and coordinated international action. Brookings Institute. Retrieved from https://www.brookings.edu/research/accelerating-the-low-carbon-transition-the-case-for-stronger-more-targeted-and-coordinated-international-action/
Ward, P. J., Jongman, B., Weiland, F. S., Bouwman, A., van Beek, R., Bierkens, M. F., ... & Winsemius, H. C. (2013). Assessing flood risk at the global scale: Model setup, results, and sensitivity. Environmental Research Letters, 8(4), 044019. https://doi.org/10.1088/1748-9326/8/4/044019
Watts, N., Amann, M., Arnell, N., Ayeb-Karlsson, S., Belesova, K., Berry, H., ... & Costello, A. (2019). The 2019 report of The Lancet Countdown on health and climate change: Ensuring that the health of a child born today is not defined by a changing climate. The Lancet, 394(10211), 1836-1878. https://doi.org/10.1016/S0140-6736(19)32596-6/